Machine Learning: when Machines Learn by Themselves
Machine Learning is the best tool for analysing, understanding and identifying patterns in data. Let us take a detailed look at how it works, how it is used in various industrial domains, and what growth is expected in the future
The term “Machine Learning” was first used in 1959 when Arthur Samuel, a pioneer of artificial intelligence (AI), defined machine learning as “a field of study that gives computers the ability to learn without being explicitly programmed”. Machine learning is therefore an application of artificial intelligence which allows a program to learn from experience and improve itself.
The idea behind Machine Learning is that a computer can be trained to automate tasks which would be tedious, difficult or impossible for a human being. In other words, Machine Learning aims to make machines more similar to us in their behaviour and decision-making process, so that they can learn from our experiences and improve. In other words, Machine Learning aims to make machines more similar to us in their behaviour and decision-making process, giving them the ability to learn from experience with minimal human intervention, that is, without any explicit programming. Virtual Assistants are an example of this.
Data are the ‘’fuel’’ for machine learning, and without data it would be actually impossible. Consequently, machine learning needs enormous computing power, data and devices capable of storing a very large amount of data. Today we have all these requirements, and we can use Machine Learning in many applications.

Machine Learning and Artificial Intelligence
Machine learning is a part of artificial intelligence which evolved from pattern recognition and computational learning. It includes the study and construction of algorithms combined with the ability to predict data.
The way a machine learns is similar to that of a human being. Humans learn from experience: the more we know, the more easily we can predict. When we face an unknown situation, the probability of success is lower than in a known situation. Machines are trained in the same way. To make an accurate prediction, the machine sees an example. When we provide it with such an example, it can reach the result automatically. However, like a human being, if it receives an example it has never seen before, it finds it difficult to make predictions.
For example, everyone knows about the Google Car, a car with a laser on the roof which tells it where it is in relation to the surrounding area. It has a radar on the front, which informs it about the speed and movement of all the vehicles around it. The Google Car uses all these data to figure out not only how to proceed, but also to predict what surrounding vehicles will do, processing almost a gigabyte of data per second.
Applications of Machine Learning technologies include for example OCR (optical character recognition), computer vision, email filtering and detecting intruders in a network. Machine Learning technology also has various applications in many industrial segments. For example, in forecasting future sales to optimise the production process, in product quality control (Quality 4.0) and in Predictive Maintenance.
Going a step further, Deep Learning, a variant of Machine Learning, has provided robots and machines with new capabilities of image and trend recognition, prediction and intelligent decision making. At the same time, the ability to predict consumer behaviour will facilitate growth in the marketing and advertising segment. In the BFSI (Banking, Financial Services and Insurance) segment, it can be widely used for wealth management, loan approval and other procedures. Added to this are segments such as document management, publishing and security.

Learning and inference
A machine learning model learns from the historical data it is provided with, and then creates algorithms to predict the output for the new data set arriving as input to the system. The accuracy of these models depends on the quality and quantity of the input data. A large amount of data will help build a better model which predicts the output more accurately.
Let us suppose we have a complex problem that requires some predictions to be made. Instead of writing code, the problem can be solved by providing data to machine learning algorithms. With their help, the machine will develop the required logic and predict the output.
The main objectives of machine learning are to learn and infer. First, the machine learns through the discovery of patterns contained in the data. A crucial role of the data scientist is therefore to carefully choose the data to be provided to the machine. The list of attributes used to solve a problem is called a feature vector, a subset of data used to address a problem.
The machine then uses certain algorithms to transform the detected patterns into a model. Basically, the learning phase is used to analyse the available data, detect the patterns present and summarise them into a model. Once the model is built, new data go through the model and its feature vector, and allow a prediction to be made. There is no need to update the rules or recalculate the model: the available model can be used to make inferences about the new data.
Thanks to Machine Learning, a machine can therefore be trained to translate an expert’s knowledge into automatic functions. Large volumes of data are usually needed to enable the machine to master a certain topic. At the beginning of its learning, the machine makes mistakes, much like a novice expert would. However, when the machine has seen a sufficient number of examples, it has acquired sufficient knowledge to work autonomously with precision.
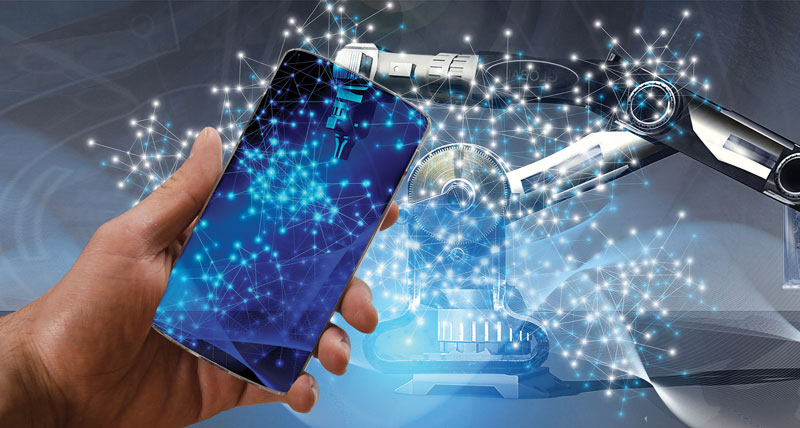
The market in the coming years
Leading organisations around the world are now using Machine Learning-based solutions to improve customer experience, ROI and obtain a competitive advantage in business operations. According to Markets and Markets, the machine learning market is expected to grow from 1.03 billion dollars in 2016 to 8.81 billion dollars by 2022, with a compound annual growth rate (CAGR) of 44.1% during the forecast period.
On the other hand, according to Cision, the global machine learning market will reach 96.7 billion dollars by 2025, with a CAGR of 43.8% from 2019 to 2025. North America has dominated the global market in recent years owing to the increasing adoption of Machine Learning technologies by major IT service providers residing in the region. However, the Asia-Pacific region is expected to record the fastest growth in the coming years, especially because of the increasing use of machine learning technologies in emerging countries such as India and China.
In the coming years, applications of machine learning in various vertical sectors are expected to increase exponentially due to new possibilities offered by technological advancements and proliferation in data generation. The need for supply chain optimization coupled with the emergence of digital services and products to enhance customer experience will drive the market growth.
Demand for Machine Learning skills is also showing strong growth, as can be seen from open positions on LinkedIn. Rising from 44,864 job openings in 2020 to 78,372 in 2021 in the US alone, organisations continue to recruit staff to quickly support new initiatives in this field. Globally, LinkedIn open positions requiring Machine Learning skills have grown from 98,371 in 2020 to nearly 200,000 in the first part of 2021.